

 |
 |
 |
 |
 |
 |
 |
 |
 |
 Information Processing Volume II: The Maximum Entropy Principle
 |
 |
 |
 |
 |
 |

 |
 |
 |
 |
 |
 |

Publisher: | Third Millennium Inferencing (Pensacola, FL) |
|  | 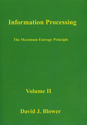 |
 |
 |
 |
 |
 |
 |
 How does an Information Processor assign legitimate numerical values to probabilities? One very powerful method to achieve this goal is through the Maximum Entropy Principle. Let a model insert information into a probability distribution by specifying constraint functions and their averages. Then, maximize the amount of missing information that remains after taking this step. The quantitative measure of the amount of missing information is Shannon's information entropy. Examples are given showing how the Maximum Entropy Principle assigns numerical values to the probabilities in coin tossing, dice rolling, statistical mechanics , and other inferential scenarios. The Maximum Entropy Principle also eliminates the mystery as to the origin of the mathematical expressions underlying all probability distributions. The MEP derivation for the Gaussian and generalized Cauchy distributions is shown in detail. The MEP is also related to Fisher information and the Kullback-Leibler measure of relative entropy. The initial examples shown are a prelude to a more in-depth discussion of Information Geometry.
 |
 |
 |
 |
 |
 |

 |
 |
|
 |
 |
 |
 |
| | | |  | |
|